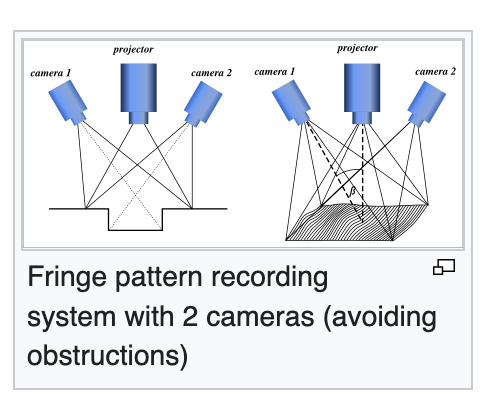
.
-as of [19 OCTOBER 2024]-
.
a structured-light 3D scanner is a device that measures the three-dimensional shape of an object by projecting light patterns—such as grids or stripes—onto it and capturing their deformation with cameras
This technique allows for precise surface reconstruction by analyzing the displacement of the projected patterns, which are processed into detailed 3D models using specialized algorithms.
Due to their high resolution and rapid scanning capabilities, structured-light 3D scanners are utilized in various fields, including industrial design, quality control, cultural heritage preservation, augmented reality gaming, and medical imaging. Compared to 3D laser scanning, structured-light scanners can offer advantages in speed and safety by using non-coherent light sources like LEDs or projectors instead of lasers. This approach allows for relatively quick data capture over large areas and reduces potential safety concerns associated with laser use. However, structured-light scanners can be affected by ambient lighting conditions and the reflective properties of the scanned objects.
Projecting a narrow band of light onto a three-dimensionally shaped surface produces a line of illumination that appears distorted from other perspectives than that of the projector, and can be used for geometric reconstruction of the surface shape (light section).
A faster and more versatile method is the projection of patterns consisting of many stripes at once, or of arbitrary fringes, as this allows for the acquisition of a multitude of samples simultaneously. Seen from different viewpoints, the pattern appears geometrically distorted due to the surface shape of the object.
Although many other variants of structured light projection are possible, patterns of parallel stripes are widely used. The picture shows the geometrical deformation of a single stripe projected onto a simple 3D surface. The displacement of the stripes allows for an exact retrieval of the 3D coordinates of any details on the object’s surface.
Generation of light patterns
[edit]
Fringe pattern recording system with 2 cameras (avoiding obstructions)
Two major methods of stripe pattern generation have been established: Laser interference and projection.
The laser interference method works with two wide planar laser beam fronts. Their interference results in regular, equidistant line patterns. Different pattern sizes can be obtained by changing the angle between these beams. The method allows for the exact and easy generation of very fine patterns with unlimited depth of field. Disadvantages are high cost of implementation, difficulties providing the ideal beam geometry, and laser typical effects like speckle noise and the possible self interference with beam parts reflected from objects. Typically, there is no means of modulating individual stripes, such as with Gray codes.
The projection method uses incoherent light and basically works like a video projector. Patterns are usually generated by passing light through a digital spatial light modulator, typically based on one of the three currently most widespread digital projection technologies, transmissive liquid crystal, reflective liquid crystal on silicon (LCOS) or digital light processing (DLP; moving micro mirror) modulators, which have various comparative advantages and disadvantages for this application. Other methods of projection could be and have been used, however.
Patterns generated by digital display projectors have small discontinuities due to the pixel boundaries in the displays. Sufficiently small boundaries however can practically be neglected as they are evened out by the slightest defocus.
A typical measuring assembly consists of one projector and at least one camera. For many applications, two cameras on opposite sides of the projector have been established as useful.
Invisible (or imperceptible) structured light uses structured light without interfering with other computer vision tasks for which the projected pattern will be confusing. Example methods include the use of infrared light or of extremely high framerates alternating between two exact opposite patterns.[2]
A 3D scanner in a library. Calibration panels can be seen on the right.
Geometric distortions by optics and perspective must be compensated by a calibration of the measuring equipment, using special calibration patterns and surfaces. A mathematical model is used for describing the imaging properties of projector and cameras. Essentially based on the simple geometric properties of a pinhole camera, the model also has to take into account the geometric distortions and optical aberration of projector and camera lenses. The parameters of the camera as well as its orientation in space can be determined by a series of calibration measurements, using photogrammetric bundle adjustment.
Analysis of stripe patterns
[edit]
There are several depth cues contained in the observed stripe patterns. The displacement of any single stripe can directly be converted into 3D coordinates. For this purpose, the individual stripe has to be identified, which can for example be accomplished by tracing or counting stripes (pattern recognition method). Another common method projects alternating stripe patterns, resulting in binary Gray code sequences identifying the number of each individual stripe hitting the object. An important depth cue also results from the varying stripe widths along the object surface. Stripe width is a function of the steepness of a surface part, i.e. the first derivative of the elevation. Stripe frequency and phase deliver similar cues and can be analyzed by a Fourier transform. Finally, the wavelet transform has recently been discussed for the same purpose.
In many practical implementations, series of measurements combining pattern recognition, Gray codes and Fourier transform are obtained for a complete and unambiguous reconstruction of shapes.
Another method also belonging to the area of fringe projection has been demonstrated, utilizing the depth of field of the camera.[3]
It is also possible to use projected patterns primarily as a means of structure insertion into scenes, for an essentially photogrammetric acquisition.
Precision and range
[edit]
The optical resolution of fringe projection methods depends on the width of the stripes used and their optical quality. It is also limited by the wavelength of light.
An extreme reduction of stripe width proves inefficient due to limitations in depth of field, camera resolution and display resolution. Therefore, the phase shift method has been widely established: A number of at least 3, typically about 10 exposures are taken with slightly shifted stripes. The first theoretical deductions of this method relied on stripes with a sine wave shaped intensity modulation, but the methods work with “rectangular” modulated stripes, as delivered from LCD or DLP displays as well. By phase shifting, surface detail of e.g. 1/10 the stripe pitch can be resolved.
Current optical stripe pattern profilometry hence allows for detail resolutions down to the wavelength of light, below 1 micrometer in practice or, with larger stripe patterns, to approx. 1/10 of the stripe width. Concerning level accuracy, interpolating over several pixels of the acquired camera image can yield a reliable height resolution and also accuracy, down to 1/50 pixel.
Arbitrarily large objects can be measured with accordingly large stripe patterns and setups. Practical applications are documented involving objects several meters in size.
Typical accuracy figures are:
Planarity of a 2-foot (0.61 m) wide surface, to 10 micrometres (0.00039 in).
Shape of a motor combustion chamber to 2 micrometres (7.9×10−5 in) (elevation), yielding a volume accuracy 10 times better than with volumetric dosing.
Shape of an object 2 inches (51 mm) large, to about 1 micrometre (3.9×10−5 in)
Radius of a blade edge of e.g. 10 micrometres (0.00039 in), to ±0.4 μm
As the method can measure shapes from only one perspective at a time, complete 3D shapes have to be combined from different measurements in different angles. This can be accomplished by attaching marker points to the object and combining perspectives afterwards by matching these markers. The process can be automated, by mounting the object on a motorized turntable or CNC positioning device. Markers can as well be applied on a positioning device instead of the object itself.
The 3D data gathered can be used to retrieve CAD (computer aided design) data and models from existing components (reverse engineering), hand formed samples or sculptures, natural objects or artifacts.
As with all optical methods, reflective or transparent surfaces raise difficulties. Reflections cause light to be reflected either away from the camera or right into its optics. In both cases, the dynamic range of the camera can be exceeded. Transparent or semi-transparent surfaces also cause major difficulties. In these cases, coating the surfaces with a thin opaque lacquer just for measuring purposes is a common practice. A recent method handles highly reflective and specular objects by inserting a 1-dimensional diffuser between the light source (e.g., projector) and the object to be scanned.[4] Alternative optical techniques have been proposed for handling perfectly transparent and specular objects.[5]
Double reflections and inter-reflections can cause the stripe pattern to be overlaid with unwanted light, entirely eliminating the chance for proper detection. Reflective cavities and concave objects are therefore difficult to handle. It is also hard to handle translucent materials, such as skin, marble, wax, plants and human tissue because of the phenomenon of sub-surface scattering. Recently, there has been an effort in the computer vision community to handle such optically complex scenes by re-designing the illumination patterns.[6] These methods have shown promising 3D scanning results for traditionally difficult objects, such as highly specular metal concavities and translucent wax candles.[7]
Although several patterns have to be taken per picture in most structured light variants, high-speed implementations are available for a number of applications, for example:
Inline precision inspection of components during the production process.
Health care applications, such as live measuring of human body shapes or the micro structures of human skin.
Motion picture applications have been proposed, for example the acquisition of spatial scene data for three-dimensional television.
Industrial Optical Metrology Systems (ATOS) from GOM GmbH utilize Structured Light technology to achieve high accuracy and scalability in measurements. These systems feature self-monitoring for calibration status, transformation accuracy, environmental changes, and part movement to ensure high-quality measuring data.[8]
Google Project Tango SLAM (Simultaneous localization and mapping) using depth technologies, including Structured Light, Time of Flight, and Stereo. Time of Flight require the use of an infrared (IR) projector and IR sensor; Stereo does not.
MainAxis srl produces a 3D Scanner utilizing an advanced patented technology that enables 3d scanning in full color and with an acquisition time of a few microseconds, used in medical and other applications.
A technology by PrimeSense, used in an early version of Microsoft Kinect, used a pattern of projected infrared points to generate a dense 3D image. (Later on, the Microsoft Kinect switched to using a time-of-flight camera instead of structured light.)
Occipital
Structure Sensor uses a pattern of projected infrared points, calibrated to minimize distortion to generate a dense 3D image.
Structure Core uses a stereo camera that matches against a random pattern of projected infrared points to generate a dense 3D image.
Intel RealSense camera projects a series of infrared patterns to obtain the 3D structure.
Face ID system works by projecting more than 30,000 infrared dots onto a face and producing a 3D facial map.
VicoVR sensor uses a pattern of infrared points for skeletal tracking.
Chiaro Technologies uses a single engineered pattern of infrared points called Symbolic Light to stream 3D point clouds for industrial applications
Made to measure fashion retailing
3D-Automated optical inspection
Precision shape measurement for production control (e.g. turbine blades)
Reverse engineering (obtaining precision CAD data from existing objects)
Volume measurement (e.g. combustion chamber volume in motors)
Classification of grinding materials and tools
Precision structure measurement of ground surfaces
Radius determination of cutting tool blades
Precision measurement of planarity
Documenting objects of cultural heritage
Capturing environments for augmented reality gaming
Skin surface measurement for cosmetics and medicine
Body shape measurement
Forensic science inspections
Road pavement structure and roughness
Wrinkle measurement on cloth and leather
Structured Illumination Microscopy
Measurement of topography of solar cells[9]
3D vision system enables DHL’s e-fulfillment robot [10]
3DUNDERWORLD SLS – OPEN SOURCE[11]
DIY 3D scanner based on structured light and stereo vision in Python language[12]
SLStudio—Open Source Real Time Structured Light[13]
Depth map
Kinect
Laser Dynamic Range Imager (LDRI)
Lidar
Light stage
Range imaging
Virtual cinematography
^ Borko Furht (2008). Encyclopedia of Multimedia (2nd ed.). Springer. p. 222. ISBN 978-0-387-74724-8.
^ Fofi, David; T. Sliwa; Y. Voisin (January 2004). “A Comparative Survey on Invisible Structured Light” (PDF). SPIE Electronic Imaging — Machine Vision Applications in Industrial Inspection XII. San Jose, USA. pp. 90–97.
^ “Tiefenscannende Streifenprojektion (DSFP) mit 3D-Kalibrierung”. University of Stuttgart (in German). Archived from the original on 9 April 2013.
^ Shree K. Nayar and Mohit Gupta, Diffuse Structured Light, Proc. IEEE International Conference on Computational Photography, 2012
^ Eron Steger & Kiriakos N. Kutulakos (2008). “A Theory of Refractive and Specular 3D Shape by Light-Path Triangulation”. Int. J. Computer Vision, vol. 76, no. 1.
^ Mohit Gupta, Amit Agrawal, Ashok Veeraraghavan and Srinivasa G. Narasimhan (2011). “Measuring Shape in the Presence of Inter-reflections, Sub-surface Scattering and Defocus”. Proc. CVPR.{{cite news}}: CS1 maint: multiple names: authors list (link)
^ Mohit Gupta; Shree K. Nayar (2012). “Micro Phase Shifting”. Proc. CVPR.
^ “ATOS – Industrial 3D Scanning Technology”. GOM GmbH. Retrieved 9 July 2018.
^ Walecki, W. J.; Szondy, F.; Hilali, M. M. (2008). “Fast in-line surface topography metrology enabling stress calculation for solar cell manufacturing for throughput in excess of 2000 wafers per hour”. Measurement Science and Technology. 19 (2): 025302. doi:10.1088/0957-0233/19/2/025302. S2CID 121768537.
^ “3D vision system enables DHL’s e-fulfillment robot”. The Robot Report. December 12, 2018.
^ Kyriakos Herakleous & Charalambos Poullis (2014). “3DUNDERWORLD-SLS: An Open-Source Structured-Light Scanning System for Rapid Geometry Acquisition”. arXiv:1406.6595 [cs.CV].
^ Hesam H. (2015). “DIY 3D scanner based on structured light and stereo vision in Python language”.
^ Wilm, Jakob; Olesen, Oline V.; Larsen, Rasmus (2014). “SLStudio: Open-source framework for real-time structured light”. 2014 4th International Conference on Image Processing Theory, Tools and Applications (IPTA). pp. 1–4. doi:10.1109/IPTA.2014.7002001. ISBN 978-1-4799-6463-5. S2CID 206932100.
Fechteler, P., Eisert, P., Rurainsky, J.: Fast and High Resolution 3D Face Scanning Proc. of ICIP 2007
Fechteler, P., Eisert, P.: Adaptive Color Classification for Structured Light Systems Proc. of CVPR 2008
Liu Kai, Wang Yongchang, Lau Daniel L., Hao Qi, Hassebrook Laurence G. (2010). “Dual-frequency pattern scheme for high-speed 3-D shape measurement”. Optics Express. 18 (5): 5229–5244. Bibcode:2010OExpr..18.5229L. doi:10.1364/oe.18.005229. PMID 20389536.{{cite journal}}: CS1 maint: multiple names: authors list (link)
Kai Liu, Yongchang Wang, Daniel L. Lau, Qi Hao, Laurence G. Hassebrook: Gamma Model and its Analysis for Phase Measuring Profilometry. J. Opt. Soc. Am. A, 27: 553–562, 2010
Yongchang Wang, Kai Liu, Daniel L. Lau, Qi Hao, Laurence G. Hassebrook: Maximum SNR Pattern Strategy for Phase Shifting Methods in Structured Light Illumination, J. Opt. Soc. Am. A, 27(9), pp. 1962–1971, 2010
Peng T., Gupta S.K. (2007). “Model and algorithms for point cloud construction using digital projection patterns” (PDF). Journal of Computing and Information Science in Engineering. 7 (4): 372–381. CiteSeerX 10.1.1.127.3674. doi:10.1115/1.2798115.
Hof, C., Hopermann, H.: Comparison of Replica- and In Vivo-Measurement of the Microtopography of Human Skin University of the Federal Armed Forces, Hamburg
Frankowski, G., Chen, M., Huth, T.: Real-time 3D Shape Measurement with Digital Stripe Projection by Texas Instruments Micromirror Devices (DMD) Proc. SPIE-Vol. 3958(2000), pp. 90–106
Frankowski, G., Chen, M., Huth, T.: Optical Measurement of the 3D-Coordinates and the Combustion Chamber Volume of Engine Cylinder Heads Proc. Of “Fringe 2001”, pp. 593–598
Je, Changsoo; Lee, Sang Wook; Park, Rae-Hong (2012). “Colour-stripe permutation pattern for rapid structured-light range imaging”. Optics Communications. 285 (9): 2320–2331. Bibcode:2012OptCo.285.2320J. doi:10.1016/j.optcom.2012.01.025.
Je, Changsoo; Lee, Sang Wook; Park, Rae-Hong (2004). “High-Contrast Color-Stripe Pattern for Rapid Structured-Light Range Imaging”. Computer Vision – ECCV 2004. Lecture Notes in Computer Science. Vol. 3021. pp. 95–107. arXiv:1508.04981. doi:10.1007/978-3-540-24670-1_8. ISBN 978-3-540-21984-2. S2CID 13277591.
Elena Stoykova, Jana Harizanova, Venteslav Sainov: Pattern Projection Profilometry for 3D Coordinates Measurement of Dynamic Scenes. In: Three Dimensional Television, Springer, 2008, ISBN 978-3-540-72531-2
Song Zhang, Peisen Huang: High-resolution, Real-time 3-D Shape Measurement (PhD Dissertation, Stony Brook Univ., 2005)
Tao Peng: Algorithms and models for 3-D shape measurement using digital fringe projections (Ph.D. Dissertation, University of Maryland, USA. 2007)
W. Wilke: Segmentierung und Approximation großer Punktwolken (Dissertation Univ. Darmstadt, 2000)
G. Wiora: Optische 3D-Messtechnik Präzise Gestaltvermessung mit einem erweiterten Streifenprojektionsverfahren (Dissertation Univ. Heidelberg, 2001)
Klaus Körner, Ulrich Droste: Tiefenscannende Streifenprojektion (DSFP) University of Stuttgart (further English references on the site)
R. Morano, C. Ozturk, R. Conn, S. Dubin, S. Zietz, J. Nissano, “Structured light using pseudorandom codes”, IEEE Transactions on Pattern Analysis and Machine Intelligence 20 (3)(1998)322–327
Fringe, 2005, The 5th International Workshop on Automatic Processing of Fringe Patterns, Berlin: Springer, 2006. ISBN 3-540-26037-4, 978-3-540-26037-0
en.wikipedia.org /wiki/Structured-light_3D_scanner
Structured-light 3D scanner
Contributors to Wikimedia projects18-22 minutes 3/18/2008
DOI: 10.1088/0957-0233/19/2/025302, Show Details
From Wikipedia, the free encyclopedia
.
.
*👨🔬🕵️♀️🙇♀️*SKETCHES*🙇♂️👩🔬🕵️♂️*
.
.
.
.
.
💕💝💖💓🖤💙🖤💙🖤💙🖤❤️💚💛🧡❣️💞💔💘❣️🧡💛💚❤️🖤💜🖤💙🖤💙🖤💗💖💝💘
.
.
*🌈✨ *TABLE OF CONTENTS* ✨🌷*
.
.
🔥🔥🔥🔥🔥🔥*we won the war* 🔥🔥🔥🔥🔥🔥